Escape the FUD -- watch on demand our webinar, “Quantifying AI Risk in Financial Terms,” for some guidelines on how to think through the risks posed by artificial intelligence tools and see a demo (starting at the 24-minute mark) of analyzing AI risk scenarios with FAIR™ (Factor Analysis of Information Risk) on the RiskLens quantitative risk analysis platform. The bottom-line advice here: You’ve got this if you apply the proven techniques of FAIR to this emerging risk.
Panelists for 'Quantifying AI Cyber Risk in Financial Terms'
Connie Matthews Reynolds, Founder/ CEO of ReynCon, LLC, longtime FAIR educator specializing in training small and large enterprises to respond to emerging threats in cybersecurity.
Katie Hinz, Security Risk Engineer at Netflix, one of the most advanced quantitative risk assessment programs in the world, and a center of FAIR risk management excellence.
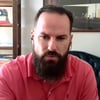
Josh Griffis, Senior Risk Consultant for RiskLens, brings extensive, up-to-date field experience in pivoting risk management programs toward AI threats.
Bernadette ‘Bernie’ Dunn, Head of Education, RiskLens, has trained over a thousand cybersecurity and risk management professionals to become FAIR practitioners.
Some highlights of the conversation...
On the state of play for AI in the cybersecurity community:
Connie Matthews Reynolds: “What I’m seeing is, a lot of people are scrambling knowing that you’re never going to be able to block it forever, and figuring out how to use it use it in a responsible way, and part of that is measuring your risk…My hope is that we can normalize what this means, build some governance, build some risk postures around individual organizations and get clarity on what does and doesn’t make sense.”
Katie Hinz: “What I am starting to see in the community is the risks that AI poses are not necessarily new risks, just a very new avenue where these risks are going to be materializing. With that context, the security community is focusing on identifying risks in terms that we know and that we are comfortable speaking in.”
Categorizing the risks from AI:
Katie Hinz: “I see it in two parts, the risks that we are receiving from generative AI as well as the risks associated with what we are putting into generative AI.
“In terms of the output we receive, organizations should understand that generative AI is not necessarily a new source of truth. It’s trained on information on the web and information we put in. So, it’s not massively different from using open-source code, for instance. Look at this in the terms of adopting any external code, with the risk of adopting malicious code or code with bugs in it. The new prong is that it’s trained on inputs so we now also face a risk of adopting intel property as our own, that would bring us to fines and judgements later.
Get hands-on experience in the RiskLens AI Risks Workshop, July 26, 11:30 AM – 3:30 PM EDT. Register now!
“In terms of what we input, a huge concern that I’m hearing, is that the data we are using to train it is very unstructured, so it’s really hard to sanitize. We’ve seen that generative AI can spit out what you’ve input to other companies…
Two things companies should want are 1) to guarantee that none of the data that employees enter into generative AI is being spread elsewhere and 2) the confidence that their employees are not blindly trusting output they receive and implementing it.”
Demo: Analyzing AI-driven risk scenarios with the RiskLens platform
As a demonstration, Josh Griffis shared the process and results of FAIR quantitative risk analysis for a hypothetical healthcare organization for two scenarios of high concern in cybersecurity:
- Breach of source code by a non-malicious insider
- Breach of sensitive data by a non-malicious insider
Josh went into detail on how he established a baseline risk of frequency and magnitude of loss events, working from existing risk data for similar previously experienced non-AI events. Then he ran through a comparison for cost vs. benefit of two mitigations: banning AI from use by company employees and improving data loss prevention (DLP) controls. His suggestion: a mix of the two – DLP upgrade plus not banning but restricting employee use – might be the most effectively solution.
Watch the webinar “Quantifying AI Risk in Financial Terms” now.
Get hands-on experience in the RiskLens AI Risks Workshop, July 26, 11:30 AM – 3:30 PM EDT. Register now!